Interpreting Yield Results – Data Variability and Evaluation
September 25, 2023
- Even the most superior seed products do not win yield comparisons in every plot.
- It is important to understand plot yield data and why a high-yielding product with the best average yield across multiple locations does not have the highest yield at every location.
- Yield data may change as the harvest season progresses and more trials are included in an overall average.
Measured yield performance of any product is the result of the genetics (G) of the product and the environment (E) in which it is tested, with the combined effects known as the G x E interaction. One must always keep in mind that yield trials deal with many variables that can contribute to yield performance. Average yields can also change as more data is accumulated across locations. Greater quantities of yield data will likely give a clearer picture of the actual yield potential.
Variability of Observations is a Natural Thing
Most genetic traits that contribute to yield are quantitative traits, which means they are controlled by multiple genes that each contribute a certain percentage to the overall trait. Observations of any quantitative trait, such as height of an individual or yield for a plot or strip trial, usually follow a bell-shaped curve (Figure 1). This variation is due to the G x E interaction, as the environment can have independent and different effects on each of the genes contributing to the expression of the trait. Most observations are close to the mean (average), but some observations—usually 5% or less—appear to be very different from the mean. These values are not wrong or incorrect, but just part of the natural variation seen in any data set. For example, consider height for men in the United States. If the average height is 70 inches, most men will be between 66 and 74 inches tall, but a few will be much taller, and a few others will be much shorter. Yield—and most other quantitative measurements of any particular trait—will follow a similar pattern.
As illustrated in Figure 1, the means of Product A and Product B are different. However, there is overlap between the two products. You can see that an individual measurement for Product B may be higher than Product A even though the overall mean of Product A is higher.
This higher measurement for Product B may be a response to the specific environment, or it may just be due to chance. Some environments may favor one product over another, resulting in a higher plot yield for Product B than for Product A even though Product A is the better overall performer. Environmental factors such as excess moisture, drought, or disease may favor Product B, while in a different environment the opposite might be true based on Product A’s and Product B’s responses to their environment. Some differences may also be due to experimental error.
The Importance of Having All the Data
Because of the inherent variability that occurs in biological systems, the initial data that is reported may not give a clear picture of the actual average performance of a product. Figure 2 charts the percent accumulated data against the rank correlations among entries in a yield plot. As harvest season begins and limited field data is collected, the correlation is low (left side of the graph area, Figure 2). As the harvest season progresses and yield data accumulates, the correlation becomes stronger and moves closer to a value of 1, with a value of 1 here representing that the true mean yield potential has been determined with 100% certainty. By the end of the harvest season, the correlation increases dramatically to over 90% (0.9 on the vertical axis, Figure 2) as the large quantity of accumulated data gives a better estimate of the yield potential of a product and its rank among the other field plot entries. Thus, with very little data accumulated, the rankings of various products within the yield plot when compared to rankings across other plots can be extremely variable. As the data is accumulated over time, how a particular product will rank in the plot will likely become more consistent and provide a better estimate of the true yield potential.
The more data that is accumulated on a given product, the more stable its ranking among multiple products becomes. In the example in Figure 1, there are eleven individual yield data points of each product, and all values are within their given distributions of the true mean yield. However, within this limited data set, seven of the eleven individual yields of Product B are closely centered or greater than the true mean yield of Product A, while only four out of eleven individual yields of Product A are greater or centered around its own true mean. While the actual mean of Product A will be greater than Product B once all the data is collected, as shown by the position of the dotted line, it would initially appear that Product B is the higher yielder based on a limited, early data set.
It is also important that when comparing products across multiple testing locations, that all the products are represented an equal number of times. For example, you may be interested in comparing the average performance of Products A, B, C and D, and you have yield results from a total of ten plot locations in your local area. If Product A appeared in only eight of the 10 trials while the Products B, C and D appeared at all 10, the data is not balanced. This unbalanced data could lead to erroneous conclusions about the performance of Product A.
Yield Stability Across Various Soil Types and Environments
As has been previously mentioned, environment (i.e., soil type, climate, irrigated or rainfed, etc.) is one of the two primary factors driving product performance relative to other products. Some products perform well in lighter-textured soil, some do not. Some perform well regardless of soil type. Placing a strip trial within a uniform soil texture is important, although with modern day yield mapping capabilities doing so has become less of an issue. However, soil texture is an example of one factor to take into consideration when selecting products. If you do not have any light-textured soil on your farm, you may not want to consider yield trials that were conducted in soils with a high percentage of sand when evaluating product performance. A certain product may have good drought tolerance which enhances its adaptation to low- or moderate-yield environments such as rainfed fields but may not be as competitive in higher-yield environments such as irrigated fields or those with high water-holding capacity.
Rolling the Test-Plot Dice
Probability is another factor in determining the winner in a test plot. The chance of a product winning depends on how many other products it is compared to in the trial, the relative difference in their yields, or the superiority of one product over another. If two products have equal yield potential, the odds of either one wining are like the 50:50 odds of head or tails when you toss a coin. If you toss a coin 10 times, you may get six heads and four tails instead of five heads and five tails, but the two outcomes are not actually significantly different. The same is true of a field trial. If two products are equal in yield potential, the odds of either one wining are 50%. If one product is superior, it will win more frequently, but a win is still not assured in every test. Figure 3 shows an example based on soybean data. Note that even if a product has a four bu/acre overall yield advantage, it will likely win only 75% of the head-to-head comparisons in a yield plot. Conversely the product that is actually four bushels less can still win 25% of the time.
Statistics 101
A statistically significant result signifies that the results are unlikely to have occurred by chance and have a high probability of being a true difference caused by trial conditions, and thus of being repeatable. If yield differences are not determined to be statistically significant, it indicates that the differences due to seed products (or other treatments) are not large enough relative to the experimental variation in the field, and that any differences are likely the result of random chance, just as when ten coinflips produce six heads and four tails.
Statistical significance is related to a number that often appears in research graphs or tables called a probability value, or p-value. This value assists in determining if the differences that are detected in a field trial are due to random error or to the treatment (e.g., seed product, fertilizer application rates, herbicide products, etc.). A common p-value for on-farm research trials is 10% (or p = 0.10). A p-value of 0.10 indicates that there is a 10% chance that any statistically significant difference among treatments is due to random chance, and a 90% chance that significant differences are due to the treatment.
Another number that university and independent plot results may include is an LSD, which stands for least significant difference. The LSD indicates the amount of yield variation that can be attributed to the product itself versus influence from outside factors. Yield differences greater than the LSD can be attributed to actual differences in genetic yield potentials of products. Yield differences less than the LSD are not considered statistically significant and are likely due to outside factors. An example is given in Table 1. Often, you will find the LSD at the bottom of the yield table in performance trial results.
Table 1. Least Significant Difference Example.
Table 1 is example data from a hypothetical university field trial whose LSD was 9 at a significance level of p = 0.10. The difference in yield between products A and B is 16 bu/acre. Because 16 is greater than the LSD of 9, we are 90% confident that the yield level is indeed significantly different and not likely due to experimental variation in the field or random chance, but to genetic differences. The difference in yield between products B and C is 8 bu/acre. Because 8 is less than the LSD of 9, we cannot conclude that the yield levels are significantly different, and the difference is likely due to experimental variation in the field or random chance, and not to genetic differences.
Summary
No product, even if it is truly superior, will win every yield plot. Over many tests, industry-leading products have typical head-to-head winning percentages of only 60 to 65%. Environmental factors, genetic potential, and test site variability constitute the variables that primarily contribute to yield differences across test plot sites. Yield ranks among entries in complied data sets can also change based on the number of tests and the geographical location of the plots. The more data and comparisons that are collected, the better the picture of yield performance. A more robust data set can increase the degree of confidence in picking a winning product.
1110_298901
Seed Brands & Traits
Crop Protection
Disclaimer
Disclaimers
- always read and follow pesticide label directions, insect resistance management requirements (where applicable), and grain marketing and all other stewardship practices. to review additional important information, click to open the menu below.expand_more
No dicamba may be used in-crop with seed with Roundup Ready® Xtend Technology, unless and until approved or specifically permitted, and no dicamba formulations are currently registered for such use in the 2024 season. Please follow www.roundupreadyxtend.com/xtendimaxupdates for status updates
Based on Court ruling and EPA Existing Stocks Order* on XtendiMax® Herbicide registration, Bayer has stopped its sale and distribution of XtendiMax® Herbicide. Visit our XtendiMax herbicide updates page to learn the latest www.roundupreadyxtend.com/xtendimaxupdates.
*Low-volatility dicamba products subject to the ruling include XtendiMax® herbicide with VaporGrip® Technology, Engenia® herbicide and Tavium® Plus VaporGrip® Technology herbicide.
The Preceon™ Smart Corn System, including short stature corn developed through traditional breeding, is expected to be available, subject to final commercialization decisions, for planting in the 2024 growing season.
Short stature corn developed through biotechnology is not currently available for commercial sale or commercial planting. Commercialization is dependent on multiple factors, including successful conclusion of the regulatory process.
This material may contain “forward-looking statements” based on current assumptions and forecasts made by Bayer management. Various known and unknown risks, uncertainties and other factors could lead to material differences between the actual future results, financial situation, development or performance of the company and the estimates given here. These factors include those discussed in Bayer’s public reports which are available on the Bayer website at www.bayer.com. The company assumes no liability whatsoever to update these forward-looking statements or to conform them to future events or developments.
*Commercialization is dependent on multiple factors, including successful conclusion of the regulatory process. The information presented herein is provided for educational purposes only, and is not and shall not be construed as an offer to sell, or a recommendation to use, any unregistered pesticide for any purpose whatsoever. It is a violation of federal law to promote or offer to sell an unregistered pesticide.
Bayer is a member of Excellence Through Stewardship® (ETS). Bayer products are commercialized in accordance with ETS Product Launch Stewardship Guidance, and in compliance with Bayer’s Policy for Commercialization of Biotechnology-Derived Plant Products in Commodity Crops. Commercialized products have been approved for import into key export markets with functioning regulatory systems. Any crop or material produced from this product can only be exported to, or used, processed or sold in countries where all necessary regulatory approvals have been granted. It is a violation of national and international law to move material containing biotech traits across boundaries into nations where import is not permitted. Growers should talk to their grain handler or product purchaser to confirm their buying position for this product. Excellence Through Stewardship® is a registered trademark of Excellence Through Stewardship.
XtendiMax® herbicide with VaporGrip® Technology has been classified as pesticide and must be used with VaporGrip® Xtra Agent (or an equivalent volatility reduction adjuvant). For approved tank-mix products (including VRAs and DRAs), nozzles and other important label information visit XtendiMaxApplicationRequirements.com. Applicators must check XtendiMaxApplicationRequirements.com no more than 7 days before application of this product for additional labeling, including state restrictions. Where applicable, users must comply with additional requirements found on this website.
ALWAYS READ AND FOLLOW PESTICIDE LABEL DIRECTIONS. Not all products are registered for use in all states and may be subject to use restrictions. The distribution, sale, or use of an unregistered pesticide is a violation of federal and/or state law and is strictly prohibited. Check with your local dealer or representative for the product registration status in your state.
Product Use Statement: Enlist E3® soybeans contain the Enlist E3 trait that provides crop safety for use of labeled over-the-top applications of glyphosate, glufosinate and 2,4-D herbicides featuring Colex-D® technology when applied according to label directions. Following burndown, the only 2,4-D containing herbicide products that may be used with Enlist® crops are products that feature Colex-D technology and are expressly labeled for use on Enlist crops. 2,4-D products that do not contain Colex-D technology are not authorized for use in conjunction with Enlist E3 soybeans. Warning: Enlist E3 soybeans are tolerant of over-the top applications of glyphosate, glufosinate, and 2,4-D. Accidental application of incompatible herbicides to this variety could result in total crop loss. When using 2,4-D herbicides, grower agrees to only use 2,4-D products that contain Colex-D technology authorized for use in conjunction with Enlist E3 soybeans. Always read and follow herbicide label directions prior to use.
YOU MUST SIGN A TECHNOLOGY AGREEMENT, READ THE PRODUCT USE GUIDE PRIOR TO PLANTING AND FOLLOW HERBICIDE RESISTANCE MANAGEMENT (HRM) REQUIREMENTS.
The transgenic soybean event in the Enlist E3® soybeans is jointly developed and owned by Corteva Agriscience and M.S. Technologies, L.L.C. ®™ Enlist, Enlist E3, the Enlist E3 logo and Colex-D are trademarks Corteva Agriscience and its affiliate companies.
Alloy™ and Connect™ are trademarks of M.S. Technologies, L.L.C., West Point, IA. Please read the M.S. Technologies, L.L.C. Use Restriction Agreement located at: - http://www.mstechseed.com/use-restriction-agreement/.
ALWAYS READ AND FOLLOW PESTICIDE LABEL DIRECTIONS. FOR COTTON TREATED FOR MARKET YEAR 2024, EACH ACCELERON® SEED APPLIED SOLUTIONS OFFERING is a combination of separate individually registered products containing the active ingredients: BASIC Offering: fluoxastrobin, prothioconazole, metalaxyl, myclobutanil, and penflufen. STANDARD Offering: fluoxastrobin, prothioconazole, metalaxyl, myclobutanil, penflufen, and imidacloprid. ELITE plus Copeo® Offering: fluoxastrobin, prothioconazole, metalaxyl, myclobutanil, penflufen, imidacloprid, and fluropyram.
FOR COTTON TREATED FOR MARKET YEAR 2022 OR PRIOR, EACH ACCELERON® SEED APPLIED SOLUTIONS OFFERING is a combination of separate individually registered products containing the active ingredients: BASIC Offering: fluxapyroxad, pyraclostrobin, metalaxyl, and myclobutanil. STANDARD Offering: fluxapyroxad, pyraclostrobin, metalaxyl, myclobutanil, and imidacloprid. ELITE plus Poncho®/VOTiVO® Offering: fluxapyroxad, pyraclostrobin, metalaxyl, myclobutanil, imidacloprid, clothianidin, and Bacillus Firmus I-1582.
Please review each seed tag to determine active ingredients in the product offering on the seed. The distribution, sale, or use of an unregistered pesticide is a violation of federal and/or state law and is strictly prohibited. Not all products are approved in all states.
ALWAYS READ AND FOLLOW PESTICIDE LABEL DIRECTIONS. FOR SOYBEANS SEED TREATMENT PRODUCTS APPLIED DOWNSTREAM, EACH ACCELERON® SEED APPLIED SOLUTIONS OFFERING is a combination of separate individually registered products containing the active ingredients: BASIC Offering: metalaxyl, penflufen and prothioconazole. STANDARD Offering: metalaxyl, penflufen, prothioconazol and imidacloprid. FOR UPSTREAM TREATED SOYBEANS, EACH ACCELERON® SEED APPLIED SOLUTIONS OFFERINGis a combination of separate individually registered products containing the active ingredients: BASIC Offering: metalaxyl, fluxapyroxad, and pyraclostrobin. STANDARD Offering: metalaxyl, fluxapyroxad, pyraclostrobin and imidacloprid. The distribution, sale, or use of an unregistered pesticide is a violation of federal and/or state law and is strictly prohibited. Not all products are approved in all states.
ALWAYS READ AND FOLLOW PESTICIDE LABEL DIRECTIONS. FOR CORN, EACH ACCELERON® SEED APPLIED SOLUTIONS OFFERING is a combination of separate individually registered products containing the active ingredients: BASIC plus Poncho®/VOTiVO® Offering for corn: metalaxyl, ethaboxam, prothioconazole, fluoxastrobin, clothianidin, Bacillus firmus I-1582. ELITE plus Poncho®/VOTiVO® Offering for corn: metalaxyl, ethaboxam, clothianidin, and Bacillus firmus I-1582; prothioconazole and fluoxastrobin at rates that suppress additional diseases. BASIC Offering for corn: metalaxyl, prothioconazole, fluoxastrobin, ethaboxam, and clothianidin. ELITE Offering for corn: metalaxyl, ethaboxam, and clothianidin; and prothioconazole and fluoxastrobin at rates that suppress additional diseases. BioRise® Corn Offering is included seamlessly across offerings on all class of 2017 and newer products. The distribution, sale, or use of an unregistered pesticide is a violation of federal and/or state law and is strictly prohibited. Not all products are approved in all states.
Performance may vary, from location to location and from year to year, as local growing, soil and weather conditions may vary. Growers should evaluate data from multiple locations and years whenever possible and should consider the impacts of these conditions on the grower’s fields.
The Bayer Carbon Program described in this material is subject to the current version of the Bayer Carbon-Smart Practices Master Agreement. The information is to aid in the understanding of the Bayer Carbon Program and does not change or modify the Bayer Carbon-Smart Practices Master Agreement in any way.
The RRXtend Spray App provides forecasts for locations within the contiguous United States. Do not use this app for forecasts outside the contiguous United States. Forecasts are for planning purposes only and are not a substitute for checking actual weather conditions at your location at the time of application and comply with the product label and other legal requirements.
B.t. products may not yet be registered in all states. Check with your seed brand representative for the registration status in your state.
Refuge seed may not always contain the DroughtGard® trait. Adament™ 50 WG Fungicide is only registered in California. Roundup Technology® includes glyphosate-based herbicide technologies.
IMPORTANT IRM INFORMATION: Certain products are sold as RIB Complete® corn blend products, and do not require the planting of a structured refuge except in the Cotton-Growing Area where corn earworm is a significant pest. Products sold without refuge in the bag (non-RIB Complete) require the planting of a structured refuge. See the IRM/Grower Guide for additional information. Always read and follow IRM requirements.
ONLY USE FORMULATIONS THAT ARE SPECIFICALLY LABELED FOR SUCH USES AND APPROVED FOR SUCH USE IN THE STATE OF APPLICATION. Contact the U.S. EPA and your state pesticide regulatory agency with any questions about the approval status of dicamba herbicide products for in-crop use with products with XtendFlex® Technology.
ALWAYS READ AND FOLLOW PESTICIDE LABEL DIRECTIONS. It is a violation of federal and state law to use any pesticide product other than in accordance with its labeling. NOT ALL formulations of dicamba or glyphosate are approved for in-crop use with Roundup Ready 2 Xtend® soybeans. NOT ALL formulations of dicamba, glyphosate or glufosinate are approved for in-crop use with products with XtendFlex® Technology.
Roundup Ready® Technology contains genes that confer tolerance to glyphosate. Roundup Ready® 2 Technology contains genes that confer tolerance to glyphosate. LibertyLink® Technology contains genes that confer tolerance to glufosinate. Roundup Ready® Flex Technology contains genes that confer tolerance to glyphosate. Roundup Ready technology contains genes that confer tolerance to glyphosate, an active ingredient in Roundup® brand agricultural herbicides. Roundup Ready 2 Xtend® soybeans contain genes that confer tolerance to glyphosate and dicamba. Products with XtendFlex® Technology contains genes that confer tolerance to glyphosate, glufosinate and dicamba. Plants that are not tolerant to glyphosate, dicamba, and/or glufosinate may be damaged or killed if exposed to those herbicides. Contact your seed brand dealer or refer to the Bayer Technology Use Guide for recommended weed control programs.
Insect control technology provided by Vip3A is utilized under license from Syngenta Crop Protection AG. Movento® MPC Insecticide is only registered for use in Florida, Georgia and Alabama. Aeris®, Balance® Flexx, Baythroid® XL, Corvus®, Degree Xtra®, Harness® Xtra 5.6L Herbicide, Harness® Xtra Herbicide, Huskie® Complete, Leverage® 360, and TriVolt™ are restricted use pesticides. Not all products are registered for use in all states and may be subject to use restrictions. The distribution, sale, or use of an unregistered pesticide is a violation of federal and/or state law and is strictly prohibited. Check with your local dealer or representative for the product registration status in your state. Fortenza Advanced™ is an on-seed application of Fortenza® and Rascendo® seed treatments. Agrisure Viptera®, Fortenza Advanced™, Fortenza® and Rascendo® are trademarks of Syngenta Group Company. Tank mixtures: The applicable labeling for each product must be in the possession of the user at the time of application. Follow applicable use instructions, including application rates, precautions and restrictions of each product used in the tank mixture. Not all tank mix product formulations have been tested for compatibility or performance other than specifically listed by brand name. Always predetermine the compatibility of tank mixtures by mixing small proportional quantities in advance. Degree® is a registered trademark of Monsanto Technology LLC. Channel®, Channel® and the Arrow Design® and Seedsmanship At Work® are registered trademarks of Channel Bio, LLC. Services and products offered by Climate LLC are subject to the customer agreeing to our Terms of Service. Our services provide estimates or recommendations based on models. These do not guarantee results. Consult with your agronomist, commodity broker, or other industry professional before making financial, farming, or risk management decisions. More information at www.climate.com/legal/disclaimer. FieldView™ is a trademark of Climate LLC. Herculex® is a registered trademark of Dow AgroSciences LLC. Clearfield®, Copeo®, ILeVO®, LibertyLink logo® , LibertyLink®, Poncho®, Poncho®/Votivo® and Votivo® are trademarks of BASF Corporation. Respect the Refuge and Corn Design® and Respect the Refuge® are registered trademarks of National Corn Growers Association. Some of the product(s) discussed herein are restricted use pesticide(s) and may not be registered in all states. The distribution, sale, or use of an unregistered pesticide is a violation of federal and/or state law and is strictly prohibited. Check with your local dealer or product representative for the product registration status in your state. Absolute®, Acceleron®, Adament™, Admire®, Aeris®, Aliette®, Alion®, Allegiance®, Asgrow and the A Design®, Asgrow®, Autumn™, Axiom®, Balance®, Bayer, Bayer Cross, Baythroid®, BioRise®, Bollgard II®, Bollgard®, BUTEO™ start, Capreno®, ConnectIN™, Corn States Triangle Design®, Corvus®, Degree Xtra®, DEKALB and Design®, DEKALB®, Delaro®, Deltapine Select™, Deltapine®, DiFlexx®, Disease Shield®, DroughtGard®, DryTough™, Emesto®, Evergol®, EverGol®, FarmFlex®, Finish®, Flint®, Fontanelle Hybrids and Design®, Fontanelle Hybrids®, Gaucho®, Gem®, Ginstar®, Gold Country Seed®, Ground Breakers®, H Hubner and Design™, Harness®, Hubner Seed & Design®, Hubner Seed Your Trustworthy Seed Resource & Design®, Hubner Seed™, Huskie®, Jung Seed Genetics & Design®, Jung Seed Genetics™, Kruger Seeds™, Laudis® , Leaf Triangle Design®, Leverage®, Lewis Hybrids and Design®, Lewis Hybrids™ , Lewis Premium Grains & Design®, Luna Experience®, Luna Privilege®, Luna Sensation®, Luna Tranquility®, Luna® Flex, Luna® Pro Fungicide, Luxxur®, MILK MAXX SILAGE RESEARCH and Design®, Minuet™, Movento®, Movento® HL, Nortron®, Oberon®, Olympus®, Poncho®, Preceon™, Proline®, Propulse®, Prosaro®, Prosper®, Provost®, Raxil®, REA Hybrids and Design™, Rea Hybrids™ , Respect the Refuge and Cotton Design®, RIB Complete and Design®, RIB Complete®, Ricestar®, Rimfire®, Roundup PowerMAX®, Roundup Ready 2 Technology and Design®, Roundup Ready 2 Xtend®, Roundup Ready 2 Yield®, Roundup Ready®, Roundup Technology®, Roundup WeatherMAX and Design®, Roundup WeatherMAX®, Roundup®, RT 3 Powered by Roundup Technology and Design®, RT 3®, Scala®, Serenade®, Serenade® SOIL fungicide, SilageSmart & Design®, SilageSmart®, Sivanto®, SmartStax®, Specialty and Design®, Specialty Hybrids™, SR and Design®, Stance®, Stewart and Design™, Stewart Seeds™, Stone Seed & Design™, Stone Seed™, Stratego®, ThryvOn™, Trecepta®, Trilex®, TripleFLEX®, TriVolt™, TruFlex™, VaporGrip®, Varro®, Velum®, Vistive®, VT Double PRO®, VT Triple PRO®, VT4PRO™, VT4PRO™ , Warrant®, WestBred and Design®, WestBred®, Wolverine®, XtendFlex®, XtendiMax® and YieldGard VT PRO® are trademarks of Bayer Group. All other trademarks are the property of their respective owners. For additional product information call toll-free 1-866-99-BAYER (1-866-992-2937) or visit our website at www.BayerCropScience.us. Bayer CropScience LP, 800 North Lindbergh Boulevard, St. Louis, MO 63167. ©2024 Bayer Group. All rights reserved.
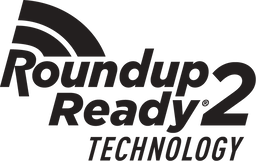
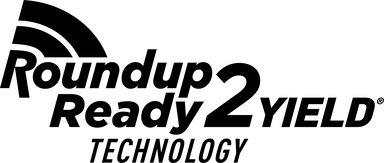
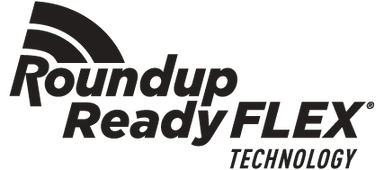
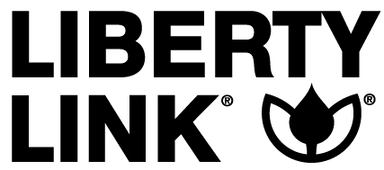
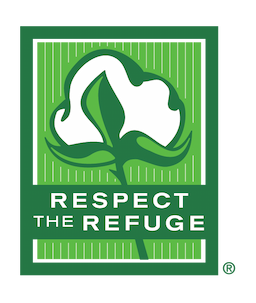
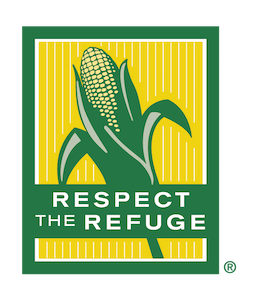
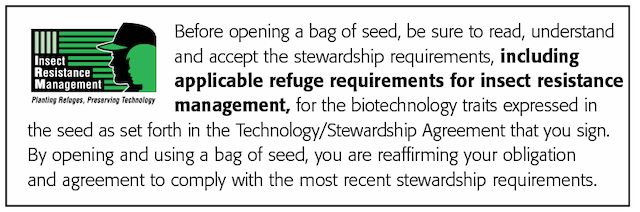
©2024 Bayer Group. All rights reserved.